Introduction
Data mining has emerged as a transformative force in the era of Big Data. By extracting valuable insights from vast quantities of information, data mining empowers organizations to make informed decisions and gain a competitive edge. As we look ahead to 2025-2030, the field of data mining is poised to undergo significant advancements, shaping industries and revolutionizing how we harness data. In this article, we explore the fascinating world of data mining and delve into what we might expect in the coming years.
The Evolution of Data Mining
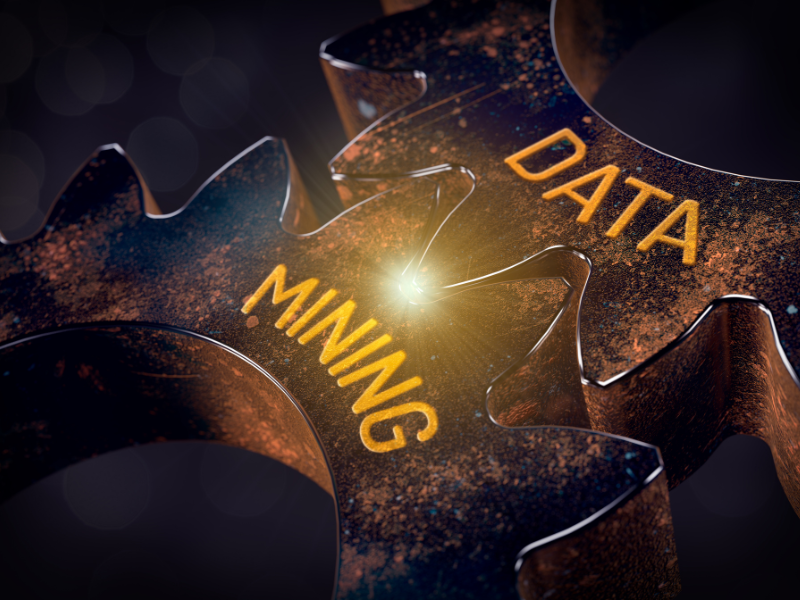
As data mining evolves, so do the techniques and methodologies employed. From its humble beginnings as a tool for descriptive analytics, data mining has evolved into a powerful mechanism for predictive analytics. By leveraging machine learning algorithms, organizations can now anticipate future trends and behaviors, providing invaluable foresight for decision-making processes.
Exploratory Data Analysis: Unleashing the Power of Visualization
Exploratory data analysis is a crucial component of data mining, enabling analysts to gain a comprehensive understanding of the underlying patterns and relationships within datasets. By leveraging visualization techniques, such as scatter plots and heat maps, data mining practitioners can unlock hidden insights that might otherwise go unnoticed.
Supervised and Unsupervised Learning: Driving Predictive Analytics
Supervised and unsupervised learning algorithms play a pivotal role in the predictive analytics capabilities of data mining. While supervised learning enables the prediction of outcomes based on labeled data, unsupervised learning uncovers hidden patterns and relationships without any predefined labels. Together, these methodologies enable organizations to make accurate predictions and drive strategic decision-making.
Ensemble Methods: Harnessing the Power of Collective Intelligence
Ensemble methods, such as random forests and boosting techniques, leverage the collective intelligence of multiple models to enhance prediction accuracy. By combining the outputs of various algorithms, data mining practitioners can mitigate the limitations of individual models and harness the true power of ensemble learning.
Advanced Techniques in Data Mining: Artificial Intelligence and Deep Learning
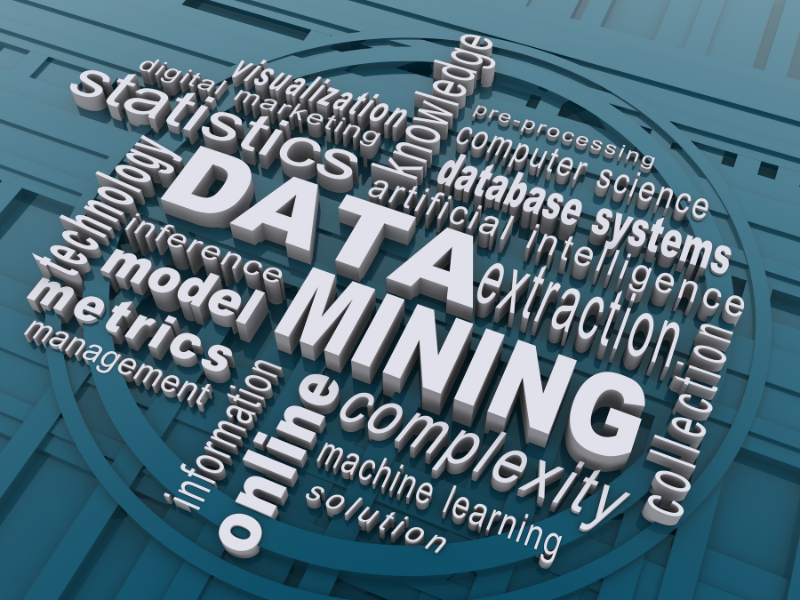
Data mining is at the forefront of embracing cutting-edge technologies, such as artificial intelligence (AI) and deep learning. As we move into the future, the convergence of AI and data mining holds tremendous potential for transforming industries and revolutionizing the way we analyze data.
Natural Language Processing: Unlocking the Power of Text Data
Natural language processing (NLP) is a subfield of AI that focuses on enabling machines to understand and interpret human language. By integrating NLP techniques into data mining workflows, organizations can unlock the wealth of insights hidden within unstructured text data, such as social media posts, customer reviews, and news articles.
Deep Learning: Decoding Complex Patterns with Neural Networks
Deep learning represents a powerful subset of machine learning that utilizes neural networks to mimic the complexity of the human brain. In data mining, deep learning algorithms excel at recognizing intricate patterns and extracting high-level features from complex datasets. As computational power increases and algorithms advance, the applications of deep learning in data mining are set to expand significantly.
Reinforcement Learning: A Paradigm Shift in Decision-Making
Reinforcement learning, a branch of machine learning, deals with training agents to make sequential decisions through a system of rewards and punishments. With applications ranging from robotics to finance, reinforcement learning has the potential to revolutionize decision-making processes in data mining, enabling organizations to optimize strategies, improve operations, and streamline processes.
Ethical Considerations in Data Mining: Striking the Balance between Innovation and Privacy
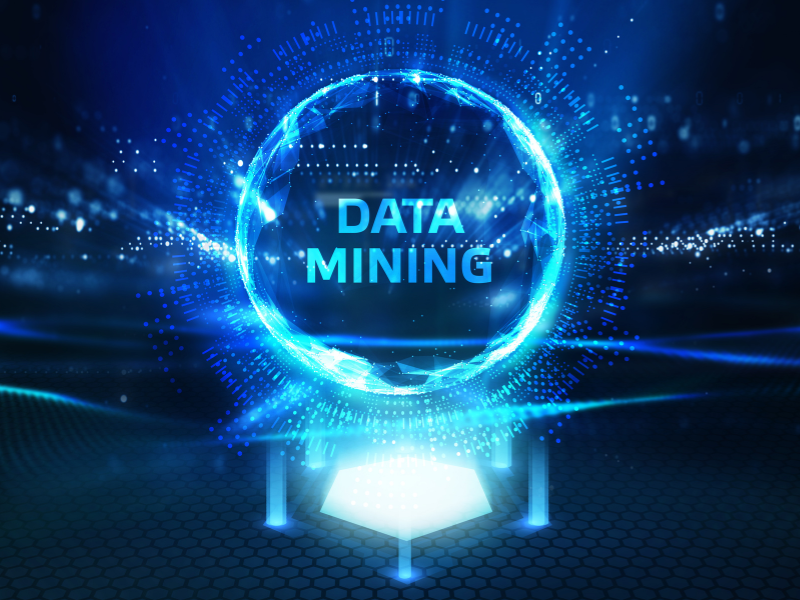
As data mining becomes increasingly prevalent, ethical considerations surrounding privacy and data usage are paramount. In the years 2025-2030, organizations must navigate the delicate balance between innovation and protecting individual rights, ensuring the responsible and ethical implementation of data mining practices.
Privacy-Preserving Data Mining: Techniques for Anonymization
Privacy-preserving data mining techniques, such as differential privacy and k-anonymity, aim to protect sensitive information while still allowing for insightful analysis. By anonymizing data, organizations can mitigate privacy concerns and ensure compliance with data protection regulations.
Bias and Fairness: Mitigating Discriminatory Outcomes in Data Mining
Data mining algorithms have the potential to perpetuate biases and discriminate against certain groups if not carefully designed and validated. Addressing issues of bias and fairness in data mining is crucial for ensuring equitable outcomes and avoiding unintended consequences. Striving for transparency and accountability in algorithmic decision-making is a key consideration for the future of data mining.
Legal and Regulatory Frameworks: Navigating the Complex Landscape
As the data mining landscape evolves, legal and regulatory frameworks must keep pace to safeguard individual rights and prevent misuse of data. Governments and policymakers play a vital role in creating robust legislation and guidelines that protect individuals’ privacy while fostering innovation and driving responsible data mining practices.
Data Mining in Industry Verticals: Transforming Domains
Organizations across various industries are leveraging data mining techniques to gain a competitive advantage and drive innovation. In the years 2025-2030, data mining is set to unleash its transformative power across numerous industry verticals, revolutionizing how we understand and harness information.
Healthcare: Enhancing Patient Outcomes through Data-Driven Insights
Data mining in healthcare holds enormous potential for improving patient outcomes, optimizing medical interventions, and guiding personalized healthcare approaches. By analyzing electronic health records, clinical data, and genetic information, data mining empowers healthcare professionals to make evidence-based decisions and deliver tailored treatments.
Finance: Risk Assessment and Fraud Detection with Data Mining
In the finance sector, data mining plays a pivotal role in risk assessment, fraud detection, and customer segmentation. By analyzing vast volumes of financial data, organizations can identify potential risks, detect fraudulent activities, and provide personalized financial services tailored to individual needs.
E-commerce: Customer Behavior Analysis for Personalization and Recommendations
E-commerce companies harness the power of data mining to gain insights into customer behavior and preferences, allowing for personalized recommendations and targeted marketing campaigns. By understanding customers’ purchasing patterns and preferences, businesses can enhance user experiences, foster customer loyalty, and drive revenue growth.
Transportation and Logistics: Optimizing Supply Chains through Data Mining
Data mining facilitates data-driven decision-making in the transportation and logistics industry, leading to more efficient supply chains, improved route planning, and enhanced delivery operations. By analyzing data from sensors, GPS devices, and historical logistics data, organizations can streamline operations and optimize resource allocation.
FAQs
Q: How can data mining improve decision-making processes? A: Data mining empowers organizations to extract valuable insights from vast amounts of data, enabling informed and data-driven decision-making. By uncovering hidden patterns and relationships, data mining helps identify trends, predict outcomes, and optimize strategies.
Q: What are the ethical considerations in data mining? A: Ethical considerations in data mining revolve around privacy, fairness, and bias. It is crucial to balance innovation with protecting individual rights, ensuring anonymity when analyzing personal data, addressing biases in algorithms, and complying with legal and regulatory frameworks.
Q: How can data mining be applied in the healthcare industry? A: In healthcare, data mining can improve patient outcomes by analyzing electronic health records, clinical data, and genetic information. It enables personalized treatment plans, optimizes medical interventions, and aids in disease surveillance and outbreak detection.
Q: Which industries can benefit from data mining? A: Data mining has applications across various industries, including healthcare, finance, e-commerce, and transportation. It facilitates risk assessment, fraud detection, personalized recommendations, supply chain optimization, and customer behavior analysis, among many other applications.
Conclusion
As we peer into the future, the potential of data mining to uncover insights and drive innovation is staggering. From advanced analytics techniques to ethical considerations, the landscape of data mining in 2025-2030 presents exciting possibilities and challenges. By embracing emerging technologies, prioritizing ethical practices, and leveraging data mining across diverse industry domains, organizations can unlock the true potential of Big Data and forge a path toward a data-driven future.